Bike race coverage has changed a lot in recent years. Gone are the days of simply sitting down to watch a basic race feed. Nowadays, we have more information at our fingertips than ever before, helping to augment the viewing experience. We have second-screen apps that allow us to track the position of every rider throughout a race, and we have on-screen data showing riders’ speed, heart rate and power output, all in real time.
Now, a group of researchers in Belgium is trying to push the envelope even further.
Steven Verstockt and Jelle De Bock are part of the IDLab research group at Ghent University, a group that uses machine learning, data mining, and computer vision techniques to help bridge the gap between data and storytelling. They’ve got projects in a number of areas but sport is of particular interest. And given De Bock is an elite cyclocross racer, it’s little surprise the research group has spent considerable effort focusing on the world of cycling.
In a conference paper written earlier this year, De Bock, Vertstockt and colleagues showed how their technology could be used to help make cycling more engaging for viewers.
At the heart of the project is metadata — effectively “data about data”. In this case that metadata is information that helps categorise or make sense of what a viewer is seeing in a race broadcast. This metadata might come from the riders themselves (via power meters, heart rate monitors and so forth), helping to better illustrate the action. Or metadata can be generated from the race broadcast feed itself, which is what the Belgian researchers are focusing on.
More specifically, they’re interested in detecting and identifying specific riders in race footage.
Pose detection
Athlete identification from video footage has been tested in other sports before (including soccer and basketball) but according to Verstockt, De Bock and colleagues, no one’s really tried this in cycling before. So how do you actually go about identifying a rider from a video feed? There are a few methods.
Image recognition of athlete numbers (think race numbers in cycling) has been tried before with a success rate of more than 94%, but it’s not a foolproof strategy — the technology only really works in shots where riders are side-on to the camera (where frame numbers are visible), or riding away from the camera (where jersey numbers are visible).
Facial recognition techniques can be used when an athlete is facing towards the camera, as an international research group showed in 2014 when analysing baseball games. This approach isn’t foolproof either though — as you’d expect, it only works for front-on shots.
To get around these shortcomings, De Bock, Verstockt and co proposed something of a hybrid strategy for rider detection, melding several techniques together. It begins with a ‘skeleton detection’ algorithm that identifies key points on a rider’s body to determine which way riders are facing in a given shot. “If the left shoulder is left of the right shoulder, then it is most likely to be a frontal shot,” the researchers explain in their conference paper. “If the left shoulder is on the right of the right shoulder, then the frame was most likely shot from a rear perspective.”
Once the algorithm knows which way the riders are facing, it can then choose which recognition module is best. “Based on the pose and the type of shot, decisions between using face recognition, jersey recognition and/or number recognition are made,” the researchers write. “Available sensor data is used to further filter or verify the set of possible candidates.”
Team and rider detection
Once the software knows what sort of shot it’s looking at, it can start to work out who is on the screen, including which teams are represented in a given shot. The researchers used a ‘Convolutional Neural Network’ — a sort of AI that can analyse visual imagery — and trained it by inputting kit photos of the 18 men’s WorldTour teams. From that, the software can look at the upper body of a rider in a shot and create a list of probabilities that the rider belongs to a given team.
The researchers write that their team detection module is currently around 80% accurate, but caveat that by saying the module was only trained on a limited dataset. They say they could improve the accuracy by feeding it more reference material, or by teaching it to recognise sponsor names on jerseys. They’re also keen to see if a separate recognition model for front, side, and rear views would be more accurate, rather than the single model currently in use.
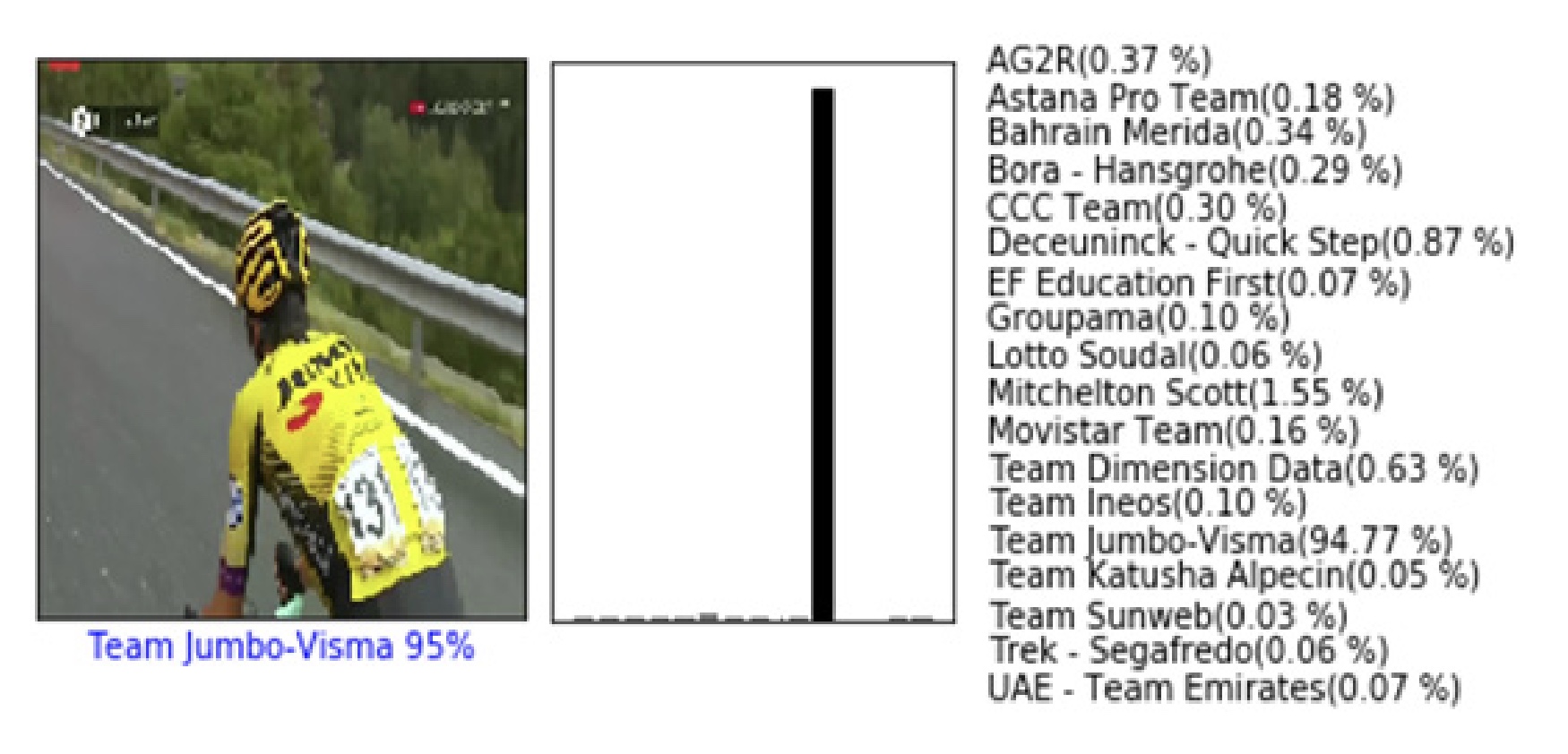
To be able to identify every rider in the race, the algorithm would need to be ‘trained’ with images of all the leaders’ jerseys, plus national, continental and world champion’s jerseys.
When it comes to identifying individual riders, the software uses one of two different modules, depending on the orientation of the shot. To identify riders from the side and rear, the software uses a text recognition module to detect a rider’s race number. When the shot is from the front, the system opts for face detection.
Face detection begins by teaching the system which faces it can expect to see. In this case the researchers used images from Wikimedia Commons which they fed into the face detection algorithm to detect and then encode the faces based on their facial features.
Face detection is already a tricky process, and in cycling it’s even trickier. “First of all, contestants always wear helmets and often sunglasses – both covering a part of their face and thus limiting the amount of ‘visible’ face features,” the researchers explain. “Second, cyclists have the tendency to look downward, rather than straight forward, which has a negative impact on performance as well.”
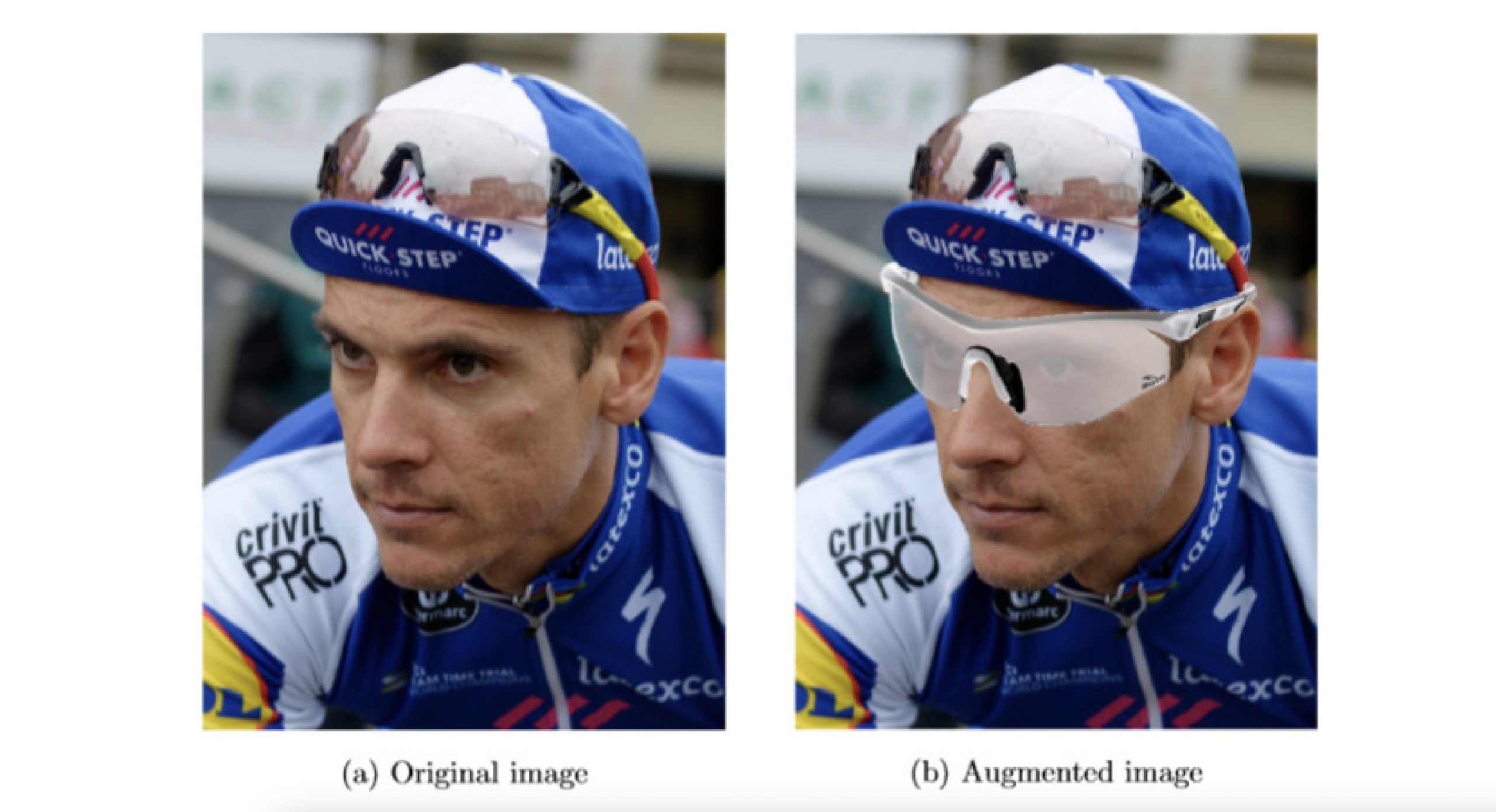
Teaching the facial identification module to recognise augmented rider images could improve accuracy when the rider is wearing glasses.
Thankfully, positional data from bike-mounted GPS trackers can help the system identify riders the recognition modules might have trouble with. Imagine a situation where a group of three riders is away from the rest of the field. If that group comes on screen, and the detection systems can identify two of those riders but not the third, working out the other rider becomes trivial.
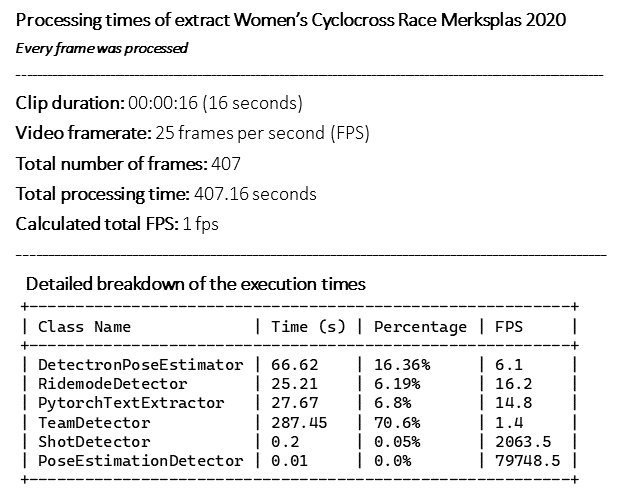
Processing times for a 16-second clip of cyclocross racing, showing how long each module takes to run.
The researchers tested their video processing pipeline on footage from a cyclocross race and from the Tour de France. They found that once their software had detected and identified a certain rider in a sample, it was able to track that rider through consecutive frames using skeleton matching.
So how fast does all this occur? The researchers explain that they are currently able to see results from around one frame per second when run in real time (i.e. for a live broadcast), “which is fine as we don’t see much improvement from processing more frames (i.e. every frame),” they tell CyclingTips via email. When they process footage after the fact — for highlights videos, say, or other edits — they use “more experimental building blocks of the pipeline. This has the advantage that we generate more metadata about the frames, but this obviously has a time cost.”
Putting it to the test
All of this is great in theory — the software can identify what sort of shot is being shown in the coverage, what riders are on screen, and it can track those riders. But what can actually be done with the resulting metadata? How can all of this be used in the real world? Well, there’s a bunch of options.
For starters, this sort of metadata can be very useful during the video production process. Automatically generating metadata showing which riders are in frame makes it considerably easier to archive and search for footage of a given rider.
There are benefits for teams too. The team detection module can determine which teams are on screen at a given moment which, in theory, should make it possible to auto-generate a video edit from a given race feed featuring only riders from that team. Similarly, it should be easy to query how much time a given team spent on TV. Such information could prove useful in sponsor negotiations.
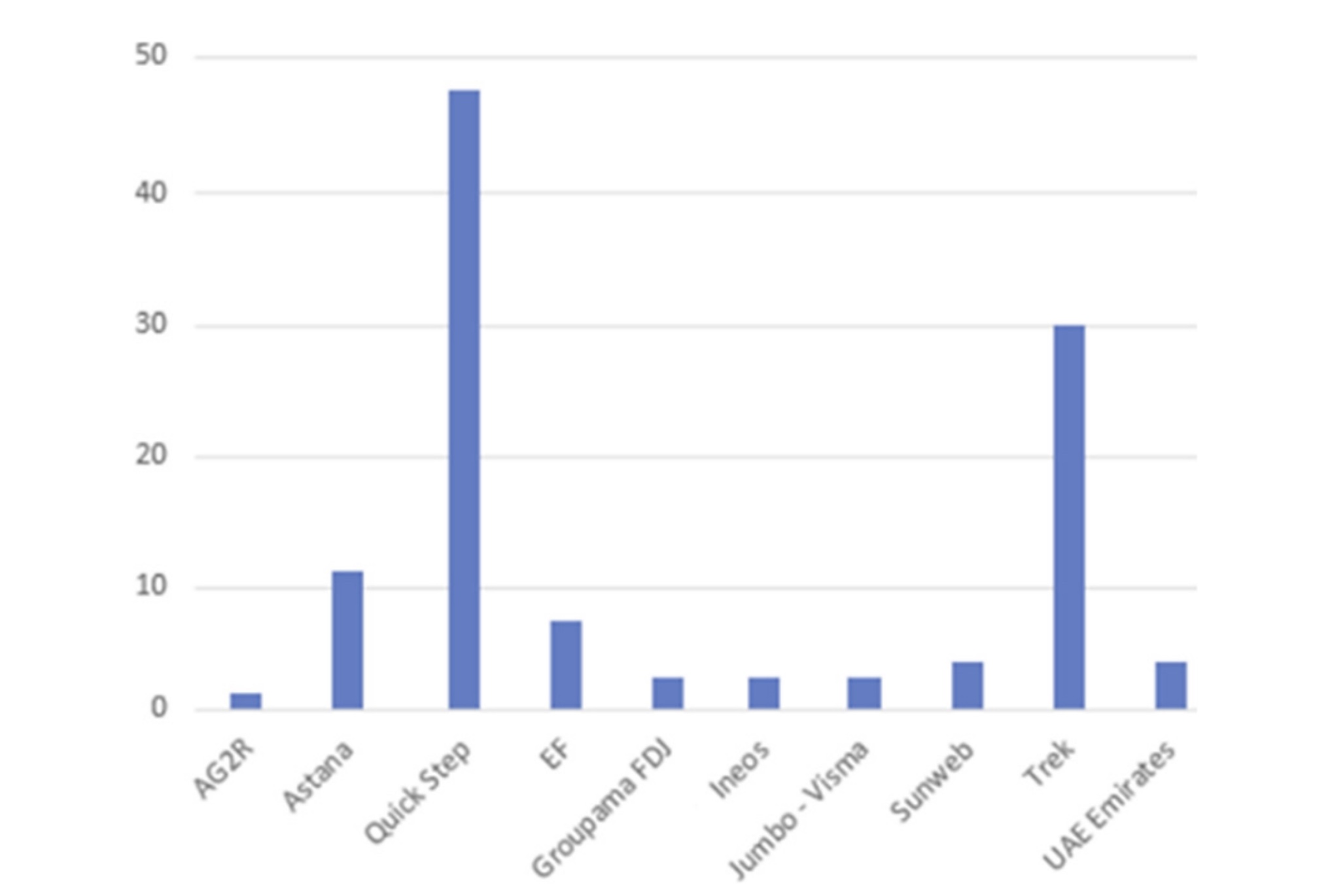
The representation of teams in footage of the final 40 km of the 2019 Clasica San Sebastian. It’s little surprise QuickStep is so well represented: Remco Evenepoel won the race solo.
Ultimately though, the researchers are most interested in the narrative potential for this technology.
“Imagine the iconic Koksijde sandy World Cup [cyclocross] course,” the researchers tell CyclingTips via email. “Michel Wuyts, one of the biggest Belgian cycling commentators often says quotes in the style of ‘I think Wout Van Aert lost the race in the first long sand sector’ or ‘Mathieu van der Poel can ride the furthest in this sector’. Of course, cycling commentators only have one pair of eyes, so they cannot see all the things that happen all of the time … This is where we want to come into play.
“With the building blocks we have, we might for instance put a fixed camera at these sand sectors and monitor the riders that ride or run the sector. These results can be processed and be provided to the audience as some interesting insights and statistics. We are currently working on an extension of the pipeline to [distinguish] between riders that are running and riders that cycle. When this data is captured and processed we should be able to ‘make or break’ the statement of commentators.
“We think that this might make the viewer experience better because commentators could use this extra knowledge to provide interesting stories about the race that otherwise would have been unnoticed.”
The following video shows this sort of application in action.
In short, De Bock and Vertstockt and co aren’t just interested in the theoretical applications of this technology — they want to see it used in the real world. From here, they’re keen to collaborate with a broadcast partner to turn their lab work into something that can benefit cycling fans.
“It will definitely still require some effort from both parties,” they admit. “Our algorithms now run in a ‘lab environment’. From what we have seen in the broadcasting industry … their current workflows are pretty much ‘closed systems’ and that even getting the race footage in real time isn’t just as easy as plugging and playing our video processing code in their video broadcasting delivery flow.
“We think that a broadcasting partner that dares to think ‘out of the box’ would be the best match to showcase what we have been doing in the lab.”